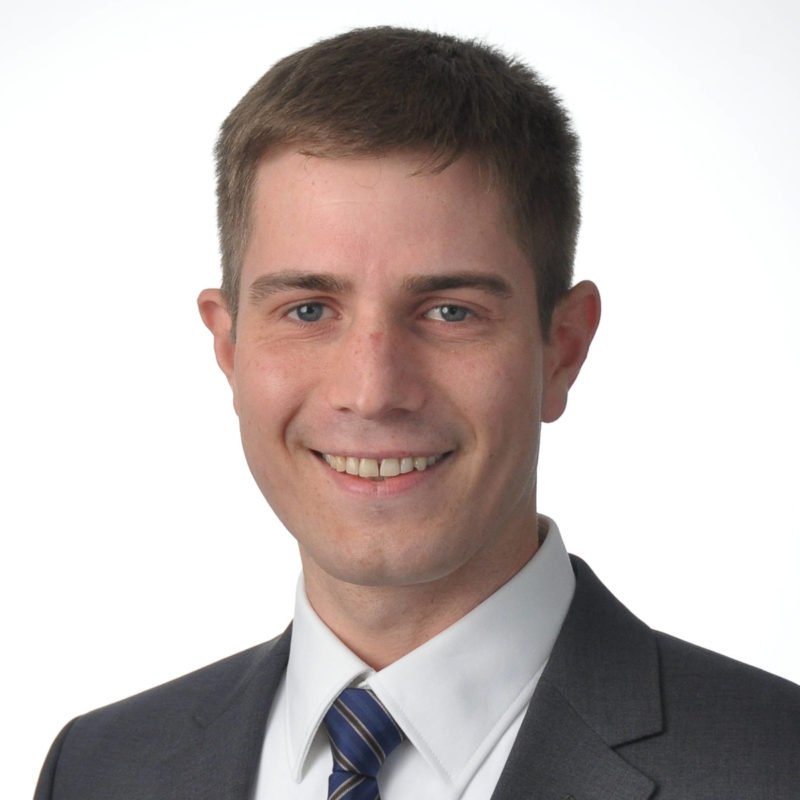
Speakers:
Dealing With the "Unknown": How We Tackle Open Set Classification Tasks at Miele
Date:
Monday, May 11, 2020
Time:
10:45 am
Summary:
Image classification has been solved successfully for many tasks e.g. using deep learning techniques. However, in many application scenarios, the set of classes from which input images are drawn is not completely known at time of modeling. This is typically the case when leaving controlled environments like in production systems and entering e.g. domestic settings. It is then of outmost importance to a) reduce false positives during model inference and b) enable the description of “unknown” image content during annotation for efficient iteration of the modeling pipeline. This case study shows how we addressed these issues at Miele for recognition of food items. Based on a comparison of state-of-the-art techniques, the presentation shows how to best reduce false positive rates. Additionally, a concept and tooling for image annotation is presented that allows to deal with “unknowns” early in the modeling process.